Project Description
In this research stream we study the social impacts of algorithmic decision-making in various domains and develop methodology to promote fairness and reliability in AI technologies. We study how biases can emerge along the machine learning (ML) pipeline, with focal points on the quality of training data, reliability and transparency in model development processes, and fairness implications of prediction-based decisions in social contexts. We further assess how ML methodology can be adopted across scientific disciplines and how AI research can benefit from social scientific and survey research perspectives.
Projects
- Aligning Generative AI Models to Human Values
- CAIUS: Consequences of AI for Urban Societies
- Evaluating Large Language Models on Linguistic Competence
- FairADM: Fairness in Automated Decision-Making
- Fair Machine Learning and Data Analysis: A Foundational Framework
- Fairness Aspects of Machine Learning in Official Statistics
- Improving Inference from Non-Random Data for Social Science Research
- Machine Learning and Causal Inference for Reliable Decision-Making in High-Stakes Settings
- Re-Evaluating the Machine Learning Pipeline to Improve Fairness and Reliability
- Refugee Integration through Algorithmic Location Matching
- Uncertainty: Sources, Quantification, & Communication
Contact Person
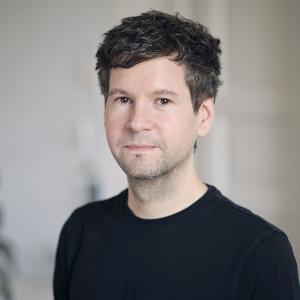
Prof. Dr. Christoph Kern
Chair of Statistics and Data Science in Social Sciences and the Humanities (SODA)