Project Description
Research suggests that the success of refugees' integration may depend on the location to which they are assigned, as specific locations may be better suited to certain characteristics of refugees (Bansak et al. (2018)). To this end, there is a growing interest in developing and deploying algorithmic refugee-location matching tools, such as GeoMatch. GeoMatch draws on supervised machine learning to predict refugee integration outcomes in potential assignment locations. Further, the tool applies an optimal matching approach to strategically assign refugees to locations where the probability of a desired integration outcome is maximised. The tool has been piloted by the Swiss State Secretariat for Migration (SEM) in Switzerland since 2020, and by the Lutheran Immigration and Refugee Service (LIRS) in the U.S. since 2023.
Although GeoMatch and similar tools, such as AnnieTM Moore, are designed to support rather than replace human decision-makers, they raise important questions about their reliability. In our research, we critically evaluate and compare existing matching tools with a focus on fairness. By assessing these tools, we aim to provide a deeper understanding of how algorithmic matching tools differ and which key components must be considered to ensure fair and reliable algorithmic decision-making in dynamic contexts.
In our empirical research, we evaluate GeoMatch for the first time in the German context by drawing on data from the German Socio-Economic Panel (SOEP).
Contact Persons
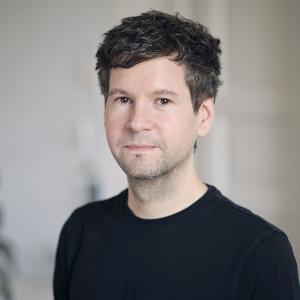
Chair of Statistics and Data Science in Social Sciences and the Humanities (SODA)
Publications
- Strasser Ceballos, C. and Kern, C. (2024). Deciding the Future of Refugees: Rolling the Dice or Algorithmic Location Assignment? Proceedings of the 3rd European Workshop on Algorithmic Fairness (EWAF’24). https://ceur-ws.org/Vol-3908/