Thesis Supervision
We provide personalized thesis supervision at both the master’s and bachelor’s levels, guiding students through the research process from the development of an idea to the final submission.
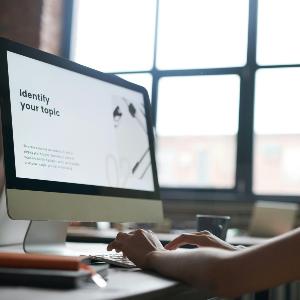
We provide personalized thesis supervision at both the master’s and bachelor’s levels, guiding students through the research process from the development of an idea to the final submission.
We supervise theses in a broad range of topics from applied to theoretical econometrics. Whether you choose to pursue a project close to our research or to explore your own ideas, we’re here to support you in developing a meaningful and rigorous thesis.
Supervisor: Mauricio Olivares, Ph.D.
Topic 1: Randomization Inference with Generated Data
The validity of the randomization tests, either in finite or large samples, relies on conditions that are easily violated with we construct the test with generated data. This happens, for example, when we want to test a hypothesis based on residuals from a linear regression. This project has a theoretical and a computational component, and the level of technicality can be adapted too.
Topic 2: Randomization Inference with Financial Data
A large body of literature in empirical finance is to determine whether there is persistence
in the performance of particular mutual funds relative to market indices. Motivated by the notion that "success breeds success"–the so-called hot-hand– one could argue that an investor could invest in funds that have performed well relative to benchmarks in recent periods. One could use recent developments in randomization inference to test this type of hypothesis in a rigorous way (Ritzwoller and Romano, 2024).
For this thesis, familiarity with randomization inference is expected. e.g., Chapter 17 of Lehmann and Romano (2023).
Topic 3: Power and Sample Size Calculations
In randomized controlled experiments, power analysis is the calculation that is used to determine the minimum sample size needed for a research study (it is conducted before the study begins). In this thesis, one could use recent developments to perform such calculations for randomization-based inference, which is particularly suitable for the analysis of randomized experiments.
Contact:
If you are interested, please get in touch with Mauricio Olivares [m.olivares@lmu.de] with your CV and a brief explanation of your interest in this topic.
Regression discontinuity (RD) designs are widely used in applied economics, finance, and political science to estimate causal effects. The methodological questions related to RD designs sparked many interesting contributions in the field of nonparametric estimation and inference. You can explore one of them in your thesis.
Supervisor: Dr. Tomasz Olma
Topic 1: Local linear estimation and inference
In practice, estimation and inference are typically conducted based on the local linear estimator combined either with robust bias corrections (implemented in the package rdrobust) or the bias-aware approach (implemented in the package RDHonest). You can explore the conclusions of different methods in an empirical application.
Topic 2: Covariate adjustments
Predetermined covariates can be used to increase the precision of the RD estimates. Various covariate adjustments have been proposed in the literature, including linear adjustments (Calonico et al., 2019), lasso-based adjustments (Kreiß and Rothe, 2023; Arai et al., 2024), and generic machine learning adjustments (Noack, Olma, and Rothe, 2024). In this project, you can compare the different approaches in simulation settings and real economic applications.
Topic 3: Extensions
You can propose your own topic related to the many extensions of the basic RD idea, such as geographic and multi-cutoff RD designs or extrapolation of treatment effects away from the cutoff.
Additional Readings:
Contact:
If you are interested, please get in touch with Tomasz Olma [t.olma@lmu.de] with your CV and a brief explanation of your interest in this topic.
Supervisor: Vincent Starck, Ph.D.
Topic 1: Analysis of diffusion in networks
Understanding the propagation of epidemics or the process of technology adoption is an important task for economics, public health, and policy. The properties of the diffusion process depend crucially on individual characteristics that influence behavior, but also on the structure of the network. A few models have been developed in the literature, each with different implications and range of applicability to real-life applications.
In this thesis, you could analyze different diffusion processes theoretically and/or via simulations, replicate some influential paper, or apply a model to a new setup.
If you are interested, please email your CV and a brief explanation your interest and familiarity with the topic to Vincent Starck [v.starck@lmu.de].